FAQs
Here we answer questions that we are frequently asked by the community.
What are research data?
The German Council for Scientific Information Infrastructures (Rat für Informationsinfrastrukturn, NFDI) has developed a generally valid definition of the term research data based on an overview of the scientific disciplines. The NFDI e.V. also refers to this definition in its “[position paper on the direct right of access to data for public research](https://www.nfdi.de/wp-content/uploads/2023/01/NFDI-Positionspapier-zum-Datenzugangsrecht-der-Forschung.pdf)”:
**research data** is not just the (final) results of research. Rather, it includes any data that is created in the course of scientific work, e.g. through observations, experiments, simulations, surveys, interviews, source research, recordings, digitisation, evaluations. Research data also includes data that has not been obtained by the researchers themselves, but which they access for research purposes in order to use it as a methodically necessary basis for the specific research process. […] The fact that the research tools used and the traces of scientific work that are created in the process – i.e. process data, as it is often automatically produced by digital research – are also considered research data is important wherever the documentation and archiving of research processes and research data is necessary for their quality assurance or for sustainability reasons or for historical research. […] Source: https://rfii.de/de/themen/
From this general definition, a specific determination of the term research data in NFDI4Objects can be derived. In the multidisciplinary research processes of our community, data is created that places numerous specific technical and conceptual demands on a community- and use-oriented infrastructure. The following data types and products are of central importance for the NFDI4Objects user community.
Primary data Object-related primary data can be the description of artefacts and their mapping, but also contexts of discovery or historical buildings.
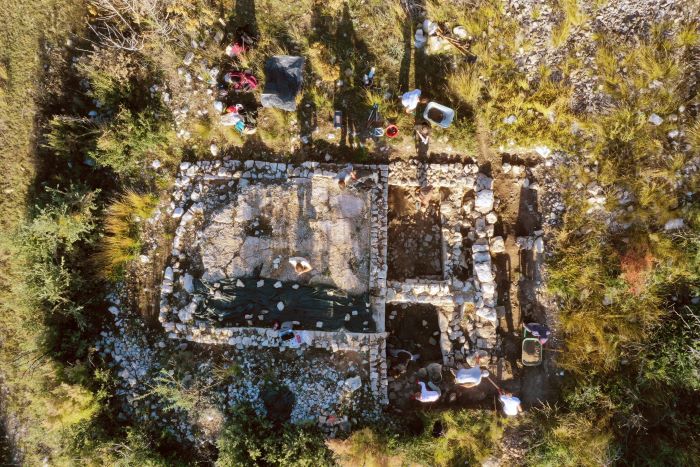
Sensor data Data from mobile, active and passive measuring sensors that are processed or generated in preparation for or in the course of documentary work in surveys and in field research.
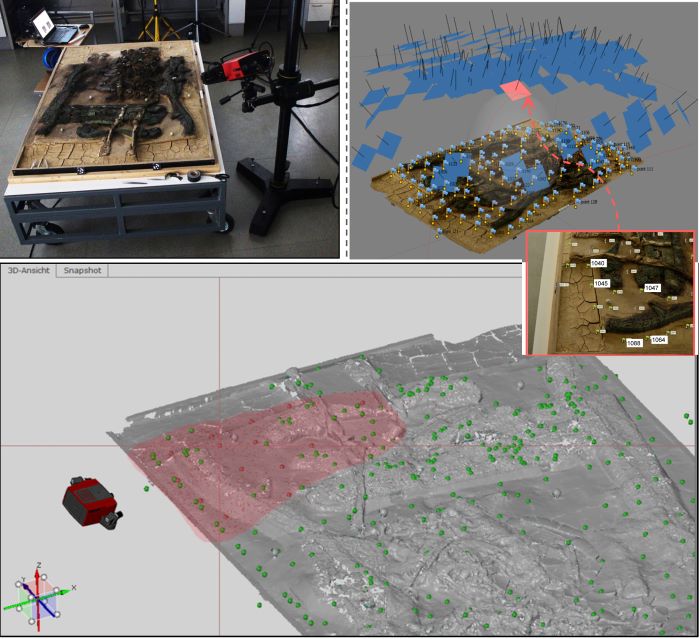
Data from laboratories Data generated in a controlled environment (laboratories) using different measurement methods.
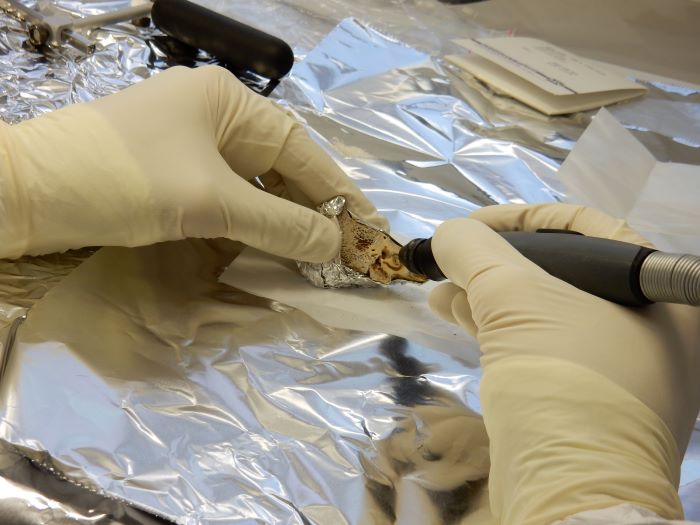
Data products Data products as a derivative of primary and laboratory data, which are prepared for use in research processes.

Legacy Data Databases, digital archives (drawings, plans, photographs) and other legacy data. This also includes local records, registers of monuments/site files, historical maps and archive data that are transferred to a digital infrastructure as part of research processes.
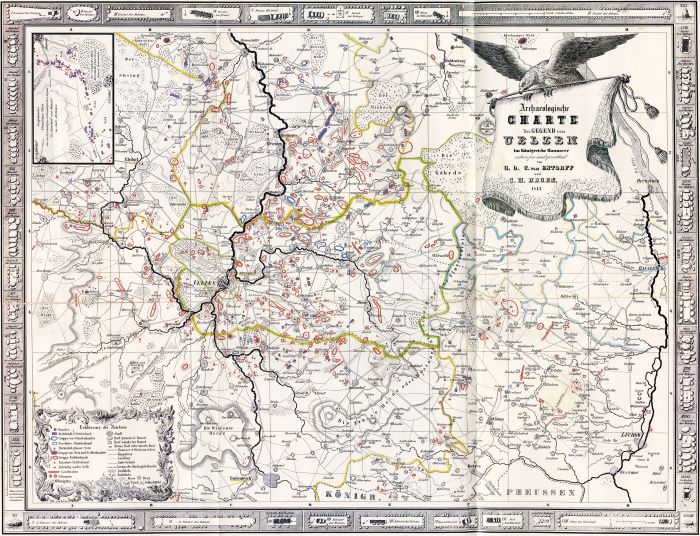
What does research data management involve?
Research Data Management (RDM) refers to the systematic and rule-based handling of research data throughout their entire life cycle. It encompasses all measures and strategies necessary to plan, generate or collect, store, organise, enrich, share and long-term archive the data.
The purpose of RDM is to ensure the traceability, reusability, quality and integrity of research results. The importance of RDM has increased dramatically in recent decades due to the sharp rise in the amount of data in science. In addition, many funding institutions make compliance with certain guidelines for handling this data a condition for the approval of research funding.
The most important aspects of research data management are:
- Data planning: Determining what data will be collected, how it will be organised and stored, and how access to it will be regulated at the beginning of a research project.
- Data organisation: Storing and organising data in a structured and well-documented way to make it comprehensible and accessible.
- Data security: Regular backups and security measures to protect data from loss or unauthorised access.
- Metadata and documentation: Creation of descriptions (metadata) that enable other researchers to understand and follow the data.
- Data archiving and sharing: Long-term storage of data in repositories and, if possible, making it available to the scientific community.
- Legal issues and ethical aspects: Taking into account data protection regulations, copyrights and ethical guidelines when handling research data. Research data management ensures that the data of a research project remains accessible, understandable and usable in the long term, which is particularly important in interdisciplinary and collaborative research projects.
What is FAIR data?
FAIR data adheres to the FAIR principles, which stand for Findable, Accessible, Interoperable and Reusable. These principles help to ensure that data is well organised and easily accessible.
Findable means that data is easy to find. This includes providing clear and unambiguous descriptions (metadata) and storing it in searchable databases.
Accessible means that data is easily accessible. It must be clear how to access the data and what the rules are for doing so.
Interoperable means that data is in a format that can be used by different systems and programmes. This is often ensured by application programming interfaces (APIs). Data should be structured in such a way that it can be combined with other data and shared.
Reusable means that data is well documented and organised so that it can be reused by others for different purposes. This includes a clear description of how the data was created and under what conditions it may be used. It also includes the aspect of long-term storage of the data in a central location. In summary, the FAIR principles help to ensure that research data is more accessible and usable in the long term for everyone.
What are the advantages of research data management for me as a scientist in object-related research?
Good research data management and a corresponding research data infrastructure offer scientists a variety of advantages that make the research process more efficient, transparent and sustainable. The specific advantages can be summarised in several areas.
-
Long-term data preservation and archiving
- Archaeological and object-related data are often unique, as excavations are unrepeatable and the contexts of finds are destroyed by the excavation itself. Good digital research data management ensures that data is stored and archived for the long term so that it remains accessible to future generations of researchers.
- Standardised data archiving ensures long-term availability, even as technologies or software evolve.
-
Accessibility and reusability
- A well-structured digital infrastructure enables scientists to store data centrally and access it from anywhere. This is particularly important in multidisciplinary and global disciplines such as archaeology, where research often takes place on an international level. Publishing data in public repositories promotes access for other scientists, who can access, further analyse or integrate existing data into their own projects. Open data accelerates scientific progress and enables the verification and replication of research results. Efficiency and time savings
- Good digital research data management makes it easier to find, organise and search for data. Instead of working through unstructured archives and paper-based notes, scientists can search specifically for metadata and specific data sets. This reduces the time needed to manage data and allows researchers to focus more on analysis and interpretation.
-
Increased transparency and traceability
- Well-thought-out research data management ensures that the research process is fully documented. All steps from data collection to analysis are made transparent, which increases the traceability of the research. It becomes replicable and reproducible.
- Especially in archaeology, where the find context is crucial, structured data management offers the possibility to review and understand results even years later. Promoting collaboration and interdisciplinarity Digital infrastructures make it easier for different research groups and disciplines to work together. Archaeologists can share their data with experts from other fields, such as anthropology, geology or genetics, to achieve more comprehensive research results. Interdisciplinary teams benefit from well-organised and interoperable data that is tagged with standardised metadata.
-
Better data quality and security
- Professional research data management can minimise errors in data processing by using tools to validate and check the consistency of data.
- Digital infrastructures usually offer automated backup mechanisms and security protocols that protect data from loss, damage or unauthorised access.
-
Fulfilling funding and legal requirements
- Many funding organisations and research institutions now require the development of a data management plan and compliance with guidelines for handling research data.
- Professional research data management helps scientists to meet these requirements and avoid problems with funding or publication.
-
Promoting scientific visibility and recognition
- By publishing data in digital repositories, scientists can gain visibility and recognition for their work because their data is accessible and citable by other researchers. Good data publication increases the reach of research and the chances of citations, which can have a positive effect on scientific reputation. Innovative research methods
- Digital research data management enables the use of new technologies and methods, such as big data analysis, artificial intelligence or 3D modelling, which are increasingly being used in object-related research. With the right infrastructure, large amounts of data can be analysed and new research questions can be asked that would not be possible with traditional methods.
-
Innovative research methods
- Digital research data management enables the sustainable use of new technologies and methods, such as big data analysis, artificial intelligence or 3D modelling, which are increasingly being used in object-related research.
- With the right infrastructure, large amounts of data can be analysed and novel research questions can be asked that would not be possible with traditional methods.
-
Ethical and legal certainty
- Research data management supports archaeologists and other researchers in complying with legal requirements (e.g. copyright, data protection, protection of cultural property) and ethical requirements, especially when dealing with sensitive data such as human remains or culturally significant finds.
- Accurate documentation of the origin and use of data protects against legal problems and ensures that research is ethically sound.
In summary, it can be said that good research data management and a functioning research data infrastructure offer considerable advantages to researchers in archaeology and related disciplines with object-related research. They facilitate the management of complex and heterogeneous data sets, promote the reusability and exchange of data, increase the efficiency of research and contribute to the long-term safeguarding of valuable data. Overall, these systems support the quality and traceability of research and enable innovative approaches to object-related research.
What is the difference between Open Data and FAIR Data?
The terms Open Data and FAIR Data are often confused because they have some overlaps. In the following, we have summarised the differences between Open Data and FAIR Data for you.
Open data means that the data is freely accessible and can be used, redistributed and modified without restriction. The data can be used by the general public at any time. The main idea behind open data is to make information freely accessible in order to promote transparency, innovation and collaboration.
FAIR stands for Findable, Accessible, Interoperable and Reusable. These principles ensure that data is well organised, structured and documented so that it can be easily found, accessed, used interoperably and reused sustainably. But FAIR data does not necessarily have to be free or accessible without restrictions. It is about clearly defining how the data can be accessed and ensuring that it is technically and semantically designed so that it can be easily integrated and used. The aim of FAIR data is to design data in such a way that it can be optimally used for scientific purposes, which promotes reusability and collaboration in research. In summary, open data emphasises the free and unrestricted use of data. Meanwhile, FAIR data describes the organisation and structuring of data to maximise its usability and reusability, regardless of whether the data is openly or restrictedly accessible.
FAIR data can also be open data, but this is not mandatory. At the same time, open data is not necessarily FAIR. The focus of FAIR is on improving the quality and usability of data, while open data is mainly aimed at accessibility.
Do you have any further questions? Then please feel free to contact our helpdesk.